Research
Vision and Goals
The South Florida Coastal Environmental Data and Modeling Center at FIU aims at developing and promoting AI/ML for coastal environmental science (ES) in Southeast Florida. We expect the Center will become a data clearing house for scientists in the environmental fields for data collection, curation, analysis, and dissemination. The Center will focus on expanding AI/ML research, particularly in trustworthy AI/ML techniques, to help understand and predict key processes affecting the ocean, urban, agricultural, and natural systems in coastal Southeast Florida. The Center will provide domain expertise and modeling support for research scientists and other stakeholders.
The Center’s specific objectives include: 1) developing a coastal environmental data management and data sharing mechanism to facilitate AI/ML research; 2) developing trustworthy AI/ML techniques with the goal of improving model performance, accuracy, and reliability, specifically for coastal environment science; and 3) applying trustworthy AI methods for coastal environmental studies specific to South Florida. Toward these goals, the Center’s research and development activities will be threefold:
- A crosscutting thrust on coastal environmental data sharing (focusing on data curation and management for AI/ML),
- Foundational research in trustworthy AI (including physics-informed AI, robust & reliable AI, and explainable AI), and
- Use-inspired AI/ML research in coastal ES.
The research plan consists of individual and yet integrated research thrusts that will address the AI/ML challenges in coastal ES, targeting three key ES areas for South Florida: sea level rise, compound coastal flooding, and harmful algae blooms.
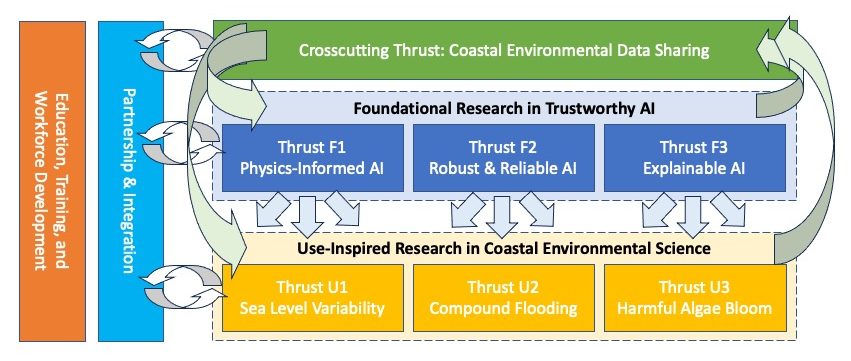
Coastal Environmental Data Sharing
Team: Liu, Crowl, Obeysekera, Bobadilla, and Tissot
FIU’s datasets cover several earth and climate science disciplines, including spatio-temporal groundwater levels, saltwater intrusion, water quality, and ocean currents. The Center will manage and make these curated datasets AI-ready. These datasets, together with the associated AI/ML applications, will be made publicly available to the research community to test AI/ML techniques and develop solutions.
Foundational Research in Trustworthy AI
Creating trustworthy AI for ES advances understanding and prediction of coastal environmental phenomena. FIU and AI2ES researchers will collaborate in this foundational research. The proposed efforts include developing physics-informed AI models by incorporating domain knowledge in the AI construction process , developing robust & reliable AI systems with noisy, corrupt, or even adversarial data and with well-calibrated uncertainty estimates, and developing explainable AI methods to analyze the spatiotemporal environmental data and understand decision processes.
Thrust F1: Physics-informed AI
Team: Dong, Bobadilla, Liu, and Tissot
Integrating physical domain knowledge into AI models can significantly reduce data requirements, as well as expedite training and prediction processes. Traditionally, AI models were manually constructed by ML expert as a black box with limited or no domain knowledge and rarely considering domain requirements. With physics-informed AI, we attend to what variables physically mean and which physical laws are driving the interpretable evolution of the analysis paradigm.
Thrust F2: Robust and Reliable AI
Team: Nguyen, Bobadilla, Luo, and McGovern
AI systems for ES applications need to be robust and reliable. They need to be robust when the number of labeled data is limited and when noisy or corrupt data is present during training. They need to provide reliable predictions with well-calibrated uncertainty estimates, since these systems are used for critical environmental applications; any errors could incur a very high cost. In this foundational AI thrust, we will develop novel AI algorithms that can achieve both robustness and reliability. We will focus on transfer learning combined with automatic denoising and uncertainty quantification techniques for environmental research.
Thrust F3: Explainable AI
Team: Luo, McGovern, Dong, and Tissot
Ensuring transparent, interpretable, and accountable decision-making processes is crucial in fostering trust in scientists who rely on these models to inform their decisions. Moreover, the interdisciplinary nature of ES necessitates the ability to comprehend and communicate AI-generated insights across a diverse range of expertise. By embracing Explainable AI (XAI) in ES, researchers can better understand the underlying mechanisms driving AI model predictions, identify potential biases, and improve model robustness. This will enhance the efficacy of AI-driven solutions in tackling critical environmental issues, ultimately empowering humanity to make more informed and responsible choices. In this thrust, we will investigate advanced XAI techniques to understand complicated environmental data and deployed machine learning models. To improve user confidence and model performance, we also propose to develop self-explainable deep learning models. Compared to traditional XAI techniques, which independently handle accuracy performance and explainability, the new paradigm aims to achieve high-performance deep learning methods with inherent interpretability.
Use-Inspired Research in Coastal ES
FIU and AI2ES researchers will apply AI/ML to coastal environmental problems specific to South Florida, including use-inspired AI/ML research in studying sea-level variability in coastal regions, compound coastal flooding due to nonlinear interactions among oceanographic, meteorological, and hydrological forces, and harmful algae blooms in Biscayne Bay.
Thrust U1: Sea Level Variability
Team: Obeysekera, He, Tissot, Dong, Luo, and Nguyen
In South Florida, frequent flooding is a challenge for residents. Coastal communities increasingly rely on real-time sea level and flood forecasting to support adaptation and resilience. Sea level variability prediction can be improved significantly by applying foundational AI research. Research tasks include:
- Physics-informed AI techniques to improve feature selection. We will utilize available simulations of ocean dynamics to expand model construction and enhance accuracy.
- Implement transfer learning. We will use transfer learning for the development of a system of spatial ML models and denoise short data records at tide gages.
- Stakeholder collaboration to implement XAI. We will use transparency, visualizations, and physical explanations to gain trust in XAI-based tools. We will get feedback and gauge trust from target users.
Trust U2: Compound Flooding
Team: Obeysekera, Tissot, Dong, Luo, and Nguyen
Compound Flooding (CF) results from nonlinear interactions among oceanographic, meteorological, and hydrological forces cause shocks and stresses on heavily urbanized coastal cities and communities. CF results from the combined effects of storm surges, waves, sea level rise, extreme rainfall, and sometimes rising groundwater tables. As an alternative to dynamical modeling, we will apply AI/ML approaches to predict CF events using both observations and simulations. Such an approach could provide trustworthy predictive tools for communities to prepare for flooding in advance. However, significant challenges remain due to lack of well-developed methods for incorporating joint probabilities of drivers (features) in AI/ML models. We will begin by developing a traditional supervised learning system to predict flooding in space and time. CF mechanisms are complex and probabilistic due to randomness but have highly correlated influencing factors, a more rigorous approach will be needed to develop CF models.
Thrust U3: Harmful Algae Bloom
Team: Boswell, He, Bobadilla, Nguyen, and Dong
Harmful algae blooms (HABs) along Florida coastlines have huge economic implications and pose severe risks to the health of residents, visitors, and aquatic life. Despite longstanding concerns about the deteriorating condition of Biscayne Bay due to nutrient runoff, limited water circulation, and increasing stagnation, we cannot predict the timing, causes, and locations of these outbreaks and their potential harm. In this thrust, we propose new AI methods to integrate collected data and first-principle models to help predict the onset of HABs and their spatiotemporal dynamics.